EXCLUSIVE: A federated analytics and AI learning platform
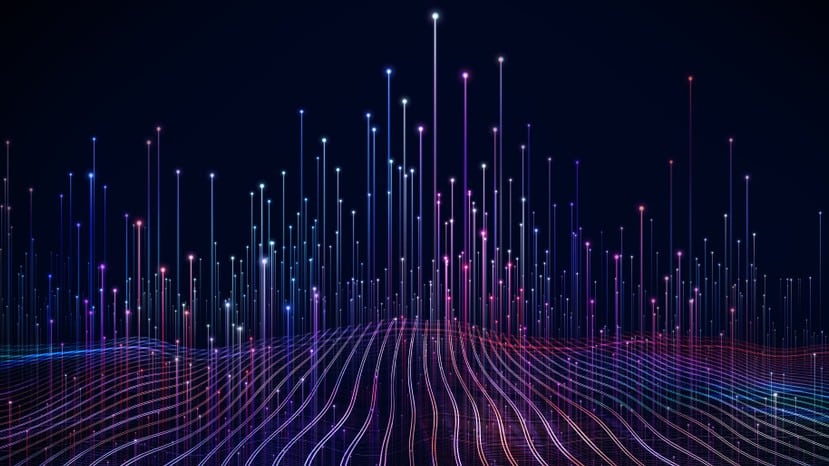
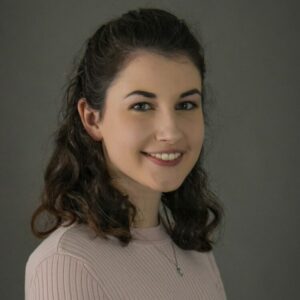
Victoria Rees
Share this content
Dell Technologies explores how an AI-based edge platform can simplify workflows and enable real-time insights.
The edge is where the digital world and physical world intersect and where data is securely generated, collected and processed to create new value. The use of edge analytics across all industries is increasing exponentially, with 80% of organizations expecting the number of AI use cases to increase in the next two years (Forrester).
With that, data science and engineering workflows and the infrastructure platforms that they run on are undergoing rapid transformation, as demand grows for more widespread automation and system autonomy.
In response, AI and machine learning (ML) are entering a third phase of development (Figure 1). In the first phase, experts defined rules for AI systems that generated descriptive and diagnostic results, mostly to identify trends. In the second, big data and the cloud provided the means to enable a more predictive approach that could help recognize the ‘why’ and ‘what’, as well as predict what will happen in the future.
Now, in this third phase, AI/ML is becoming both prescriptive and adaptive, with a wide range of intelligent edge systems delivering data-driven benefits, extending to nearly all facets of human activity. This means being able to make near-real time decisions to take advantage of an opportunity or to mitigate risk.
Most organizations that have embraced AI/ML and analytics are somewhere between the diagnostic and predictive phases, meaning they are still a long way from realizing their data maturity potential.
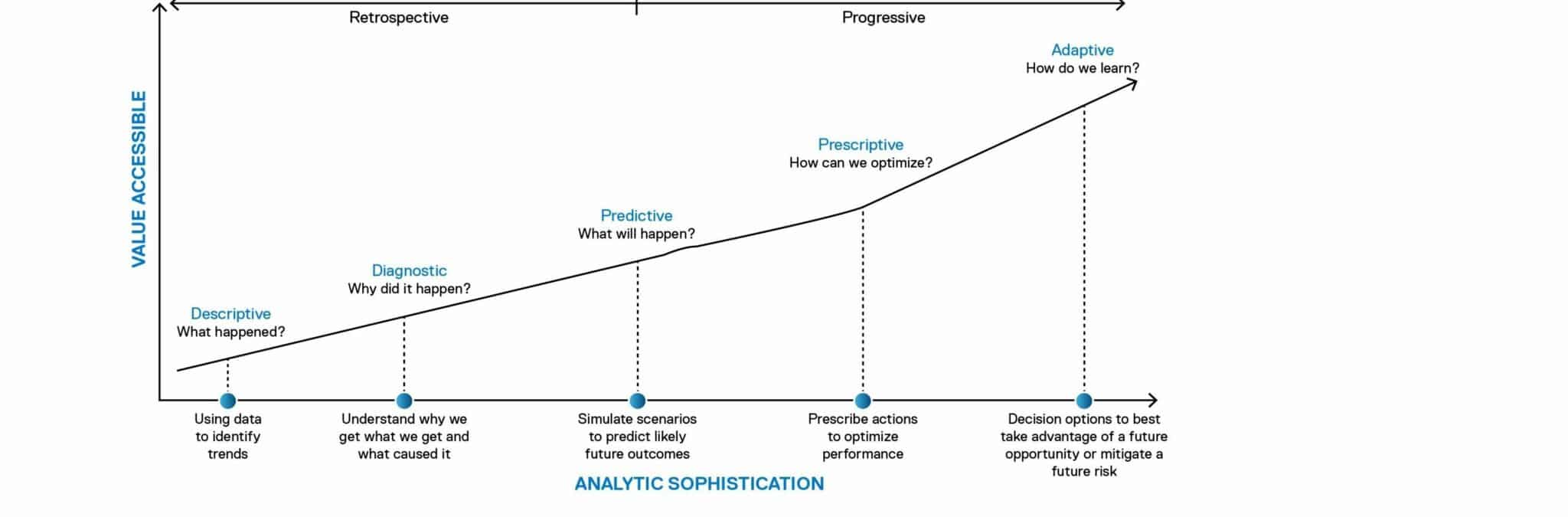
AI/ML processing at the edge
Traditional AI/ML centralized and distributed architectures were not designed to support the data demands of modern autonomous systems, particularly as the largest volume of edge data is video based. These architectures have inherent limitations in being able to effectively gather, process, correlate, analyze and utilize the information from edge devices in near real time.
For example, in a centralized framework, all data must be sent across the network to a data center or the cloud before deep learning models can generate the required insights. This poses significant drawbacks, especially when the value of data at the edge is time sensitive. A self-driving car, for example, can’t wait for the centralized AI platform to decide whether its sensors may have detected an obstacle in the road and if so, what action needs to be taken. That decision needs to be made immediately, in real time.
This is where a federated approach to AI/ML processing benefits. It allows computational processes, AI and ML algorithms to be run on data sets at the edge as they are gathered, while sharing only mathematical models, metadata and results of these queries over the network to other edge devices, data center(s) or cloud.
However, the need for intelligence where the data resides and moving computing power to the edge introduces new levels of complexity.
Key AI/ML computing and workflow challenges
Every AI/ML system requires teams of people who support the system and infrastructure for end users to derive the insights needed for business and operational outcomes. These teams tend to face unique challenges in their respective roles, including:
- The data scientists and DevOps teams in terms of creating the algorithms and workflows
- The IT and infrastructure teams, in relation to deployment, infrastructure, security and compliance challenges
- The consumers of the data, such as line of business managers, who require qualitative insights in near real time
A major challenge for data science and DevOps teams is the amount of time it takes to create and/or retrain a model. The lack of IT skills and limited AI expertise or knowledge is a key barrier and a major concern for many organizations, as is the lack of tools or platforms for developing AI models. Data accessibility is also an issue, in that AI systems are only as good as the data used to train them. Data scientists face difficulties when analyzing data to build and scale trusted AI and when collecting data to make it simple and accessible.
Moving greater computing power to edge devices situates processing closer to where data is being collected. However, this introduces new levels of complexity for IT and infrastructure teams looking to deploy AI at the edge, including:
- Network bandwidth: shifting computing and data to the edge means that endpoints utilize more bandwidth. Edge computing puts greater emphasis on having more bandwidth across the entire network, where it was previously more focused on the core
- Compute: with AI, there is a need to distribute the computing power more evenly. This means the edge infrastructure must be provisioned with enough resources. This is often overlooked and can create bottlenecks if not correctly sized
- Latency: Edge computing places the computing power closer to where the data is collected, which reduces both application latency and decision-making latency. However, the process of building learning models could still mean massive amounts of data will need to be moved back to the central core computing resource
The quality and timeliness of data insights are critical for data consumers. Data needs to be processed, analyzed and acted on in real time to be worthwhile. However, regionalization of data increases data complexity and data silos limit the quality of the insights. Also, AI cannot succeed without data that is properly prepared and curated; understanding and being able to ensure the quality of the data insights is a key obstacle.
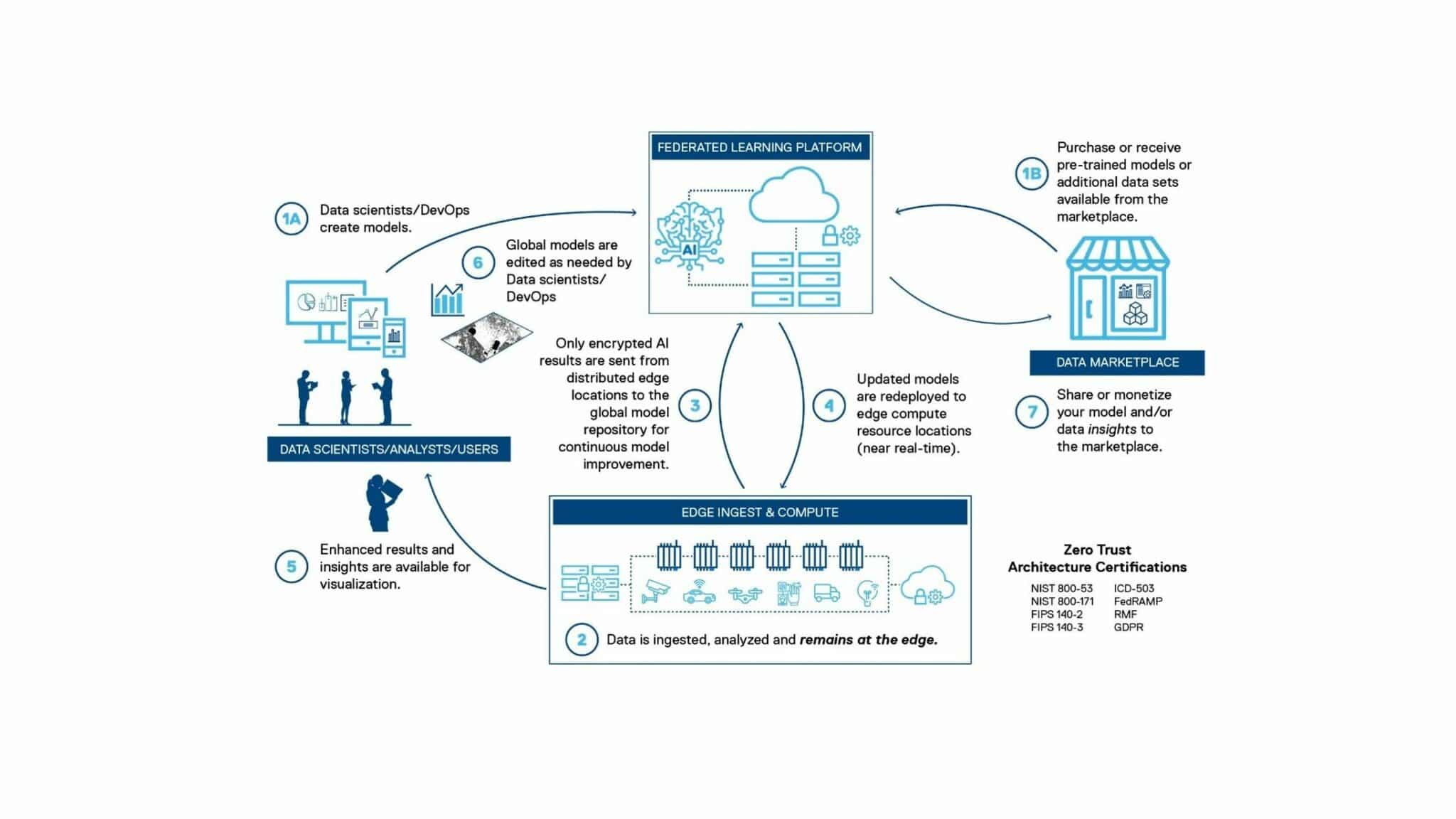
A federated analytics and learning platform
Edge computing devices can process incoming data immediately in real-time as with a distributed system and send the results of the inferencing back to the centralized training model to improve the quality of analytics.
The new optimized federated data models that incorporate results from all input sources can then be redeployed back to all the edge devices. Keeping the data local and sharing only the inferencing in the federated system enables the exchange of models and algorithms between edge devices, endpoints and even organizations.
A significant benefit of the federated platform to organizations is the built-in data science and data engineering tools, which greatly simplifies workflows, accelerates the development process and shortens the time data scientists need to execute on their vision. The low-code or no-code platform provides a client interface that data scientists and analysts can use to understand the data and generate the desired insights and dashboards based on their role in the organization. Dashboards can be easily created for any specific function being managed, for example operational, security or revenue outcomes.
There is also support for the most common open-source AI frameworks. The automation of basic AI tasks includes support for the entire lifecycle from data ingestion, training and model deployment to results visualization.
Model training takes place at the edge for network cost and privacy advantages without requiring data to be moved to a centralized lake. The accuracy of a federated system is also increased by having access to all relevant models, results and metadata that are available throughout the broader operational ecosystem – whether from other devices, the core or cloud. This more accurate model enables real-time insights for better and more timely decisions.
As demand for automation and system autonomy grows across industries, organizations are needing a platform to deliver real-time analytics with the ability to unlock the value of data to provide actionable insights and desired business outcomes. The Dell Technologies federated analytics platform combines centralized and distributed, lab-validated AI architectures to help address the challenges posed by the increase in computing at the edge as the number of sensors and data they produce proliferates.
This article was originally published in the April edition of Security Journal Americas. To read your FREE digital edition, click here.