EXCLUSIVE: From crop to shelf – how computer vision optimizes food production
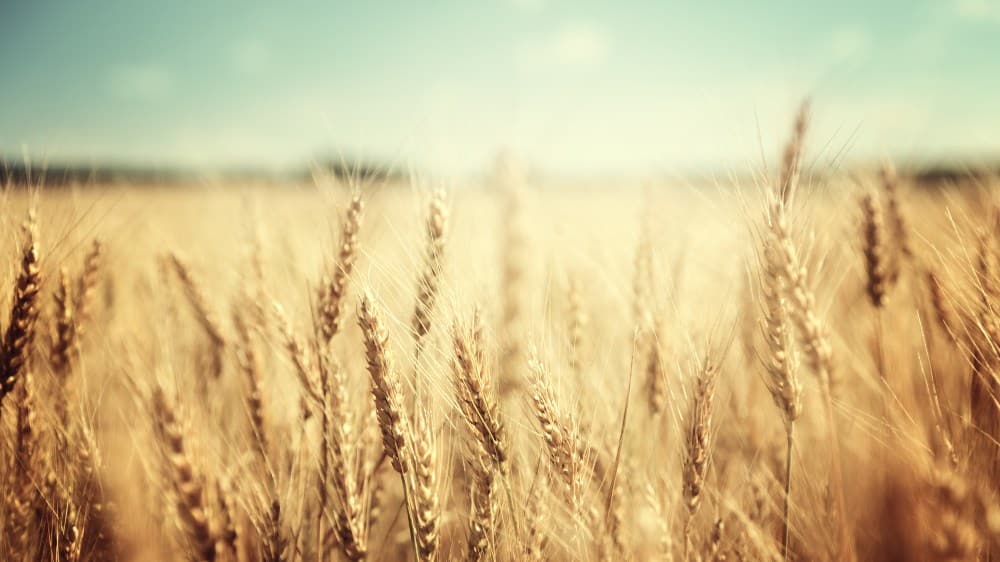
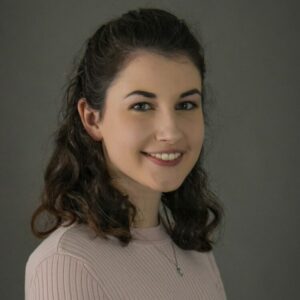
Victoria Rees
Share this content
In this case study, Dell Technologies outlines how computer vision and federated analytics can improve the availability of food.
Consider the impact of data analytics on a global food manufacturer with plants across the world and a major grocery retailer with over 10,000 locations.
The aggregation of even small efficiencies throughout a large manufacturing process accelerates production, helps the company achieve its sustainability goals and generates revenue more quickly, while a steady stream of product making it to store shelves attracts customers, increasing the retailer’s revenue.
Critical decision making is now data and computer driven, requiring not only accuracy but the capability to capture, store and access data when and where it’s needed. However, the volume of data is exploding and is causing the traditional centralization topologies to become more problematic and overloaded from the perspective of connectivity and costs.
To process data efficiently and gain business insights, a federated analytics and learning platform provides computer and data storage at the edge and in the cloud. Most data remain at the edge and only data used for training the deep learning models is uploaded to the cloud.
The food production process – a case study
Now, let’s look at a food manufacturer in more detail; the company sought to optimize its quality management processes by digitizing and streamlining business and operational processes. Part of this effort involved improving the efficiency of receipt and storage of grain at thousands of facilities. Grain intake was a paper-driven, manual process that took about 70% of operators’ time.
Using computer vision and federated analytics, the manufacturer was able to automate that process. Cameras now read truck license plates and take pictures of what is on each truck, weights are recorded and remote sensing technology helps determine the appropriate bay for each truck. Ingesting and digitizing all of this data at the edge optimized grain intake and enabled real-time reporting, improving efficiency by over 30%. Expediting the intake process also reduces pollution because trucks are idling for much less time.
The retailer experienced pains common to the industry. Ensuring employee and customer safety was a top priority. Shrink – or loss – whether from processes, employees or customers, reduced store margins significantly. Additionally, the inability to access and gain insights from disparate data prevented proactive decision making.
Each store location has an IT equipment rack that houses servers to support network operations, email and other enterprise applications. Operational technology (OT) includes surveillance cameras, digital video recorders, point of sale systems, building management systems and other non-IT technology. OT accounts for a large majority of the total data footprint for any location and the data was often siloed and required manual extraction to external media. The cameras alone totaled 150,000 across all the retail sites, each generating huge volumes of unstructured data and no way to remotely access it.
Security and theft-related events across thousands of stores require internal reporting, with legal cases created and video made available to law enforcement upon request. The number of incidents and cameras requires an enormous level of human resources dedicated to managing information and providing materials to stakeholders.
The retailer wanted to consolidate high-level data from both IT and OT to drive decisions and respond more efficiently to requests. In addition, although the retailer had in-house data scientists, the company wanted to customize off-the-shelf AI models to reduce the load on the data science and data engineering teams.
Overall, the retailer needed a common computer and storage blueprint, whether at the edge or centralized, for collecting and processing data that is right-sized and capable of scaling to fit the needs of each location. The implementation of a federated analytics and learning platform not only enabled consolidation of IT and OT data but made it accessible 24/7, as well as being easier to manage, along with the capability to search video by location and specific dates. It also enabled tighter and traceable data governance and cyber compliance.
Outcomes
With greater data-driven insights based on camera data, shrink was reduced by about 1.5% of total revenue and the safety and security of locations was improved. In addition, the solution facilitates the use of in-house and third-party models, accelerating the development process while increasing the retailer’s adoption of AI.
The retailer also realized gains in other forms of operational efficiency and sustainability. For example, the platform continuously monitors the electrical draw from refrigerator and freezer cases in the stores, notifying staff when equipment needs to be serviced, enabling predictive maintenance and minimizing power consumption.
Dell Technologies’ Federated Analytics and Learning Platform gives organizations the tools needed to easily manage and draw insights from data captured and managed at the edge. With models available through a marketplace and fully customizable, organizations do not require dedicated data science and data engineering teams to derive business insights.
By pushing only training data to the cloud, security is inherent in the platform’s design, ensuring compliance with any standard on the globe. Coupled with computer vision, customers are increasing personnel and facility safety, enhancing the customer and employee experience, improving operational efficiency, contributing to better sustainability practices and expanding revenues.
This article was originally published in the April edition of Security Journal Americas. To read your FREE digital edition, click here.